EEOB Publication - Berger-Wolf
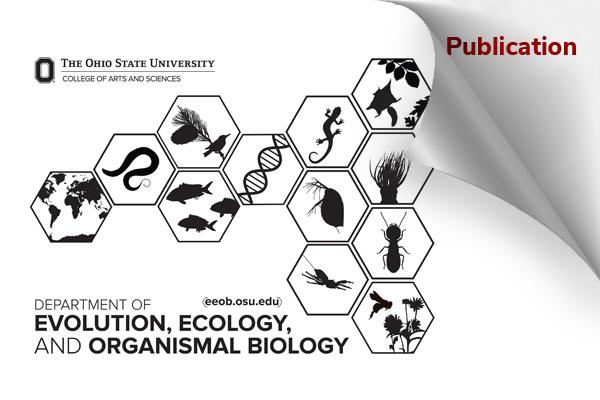
Deep dive into KABR: a dataset for understanding ungulate behavior from in-situ drone video
Maksim Kholiavchenko, Jenna Kline, Maksim Kukushkin, Otto Brookes, Sam Stevens, Isla Duporge, Alec Sheets, Reshma R. Babu, Namrata Banerji, Elizabeth Campolongo, Matthew Thompson, Nina Van Tiel, Jackson Miliko, Eduardo Bessa, Majid Mirmehdi, Thomas Schmid, Tanya Berger-Wolf, Daniel I. Rubenstein, Tilo Burghardt & Charles V. Stewart. Multimed Tools Appl (2024). https://doi.org/10.1007/s11042-024-20512-4
Abstract
In this paper, we extend the dataset statistics, model benchmarks, and performance analysis for the recently published KABR dataset, an in situ dataset for ungulate behavior recognition using aerial footage from the Mpala Research Centre in Kenya. The dataset comprises video footage of reticulated giraffes (lat. Giraffa reticulata), Plains zebras (lat. Equus quagga), and Grévy’s zebras (lat. Equus grevyi) captured using a DJI Mavic 2S drone. It includes both spatiotemporal (i.e., mini-scenes) and behavior annotations provided by an expert behavioral ecologist. In total, KABR has more than 10 hours of annotated video. We extend the previous work in four key areas by: (i) providing comprehensive dataset statistics to reveal new insights into the data distribution across behavior classes and species; (ii) extending the set of existing benchmark models to include a new state-of-the-art transformer; (iii) investigating weight initialization strategies and exploring whether pretraining on human action recognition datasets is transferable to in situ animal behavior recognition directly (i.e., zero-shot) or as initialization for end-to-end model training; and (iv) performing a detailed statistical analysis of the performance of these models across species, behavior, and formally defined segments of the long-tailed distribution. The KABR dataset addresses the limitations of previous datasets sourced from controlled environments, offering a more authentic representation of natural animal behaviors. This work marks a significant advancement in the automatic analysis of wildlife behavior, leveraging drone technology to overcome traditional observational challenges and enabling a more nuanced understanding of animal interactions in their natural habitats.